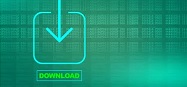
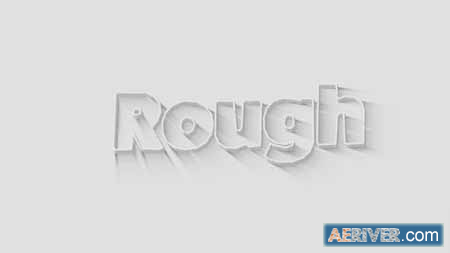
models.keyedvectors – Store and query word vectors.models.lda_worker – Worker for distributed LDA.models.lda_dispatcher – Dispatcher for distributed LDA.models.lsi_worker – Worker for distributed LSI.models.lsi_dispatcher – Dispatcher for distributed LSI.anslation_matrix – Translation Matrix model.models.logentropy_model – LogEntropy model.models.hdpmodel – Hierarchical Dirichlet Process.models.ldaseqmodel – Dynamic Topic Modeling in Python.models.lsimodel – Latent Semantic Indexing.models.nmf – Non-Negative Matrix factorization.models.ensembelda – Ensemble Latent Dirichlet Allocation.models.ldamulticore – parallelized Latent Dirichlet Allocation.models.ldamodel – Latent Dirichlet Allocation.corpora.wikicorpus – Corpus from a Wikipedia dump.corpora.ucicorpus – Corpus in UCI format.corpora.textcorpus – Tools for building corpora with dictionaries.corpora.svmlightcorpus – Corpus in SVMlight format.corpora.sharded_corpus – Corpus stored in separate files.corpora.opinosiscorpus – Topic related review sentences.corpora.mmcorpus – Corpus in Matrix Market format.corpora.malletcorpus – Corpus in Mallet format.corpora.lowcorpus – Corpus in GibbsLda++ format.corpora.indexedcorpus – Random access to corpus documents.corpora.hashdictionary – Construct wordid mappings.corpora.dictionary – Construct wordid mappings.corpora.csvcorpus – Corpus in CSV format.corpora.bleicorpus – Corpus in Blei’s LDA-C format.The dimensionality K of Dirichlet distribution (aka # of topics) is assumed to be known and fixed. Use these assignments to estimate topic mixtures of each document (\% words assigned to each topic within that document) and word associated to each topic (\% of words assigned to each topic overall).Keep iterating until assignments reach a steady state.Update assignment of current word in the loop assuming topic assignment distributions for the rest of the corpus are correct.Reassign with a new topic, where we choose topic t with probability $p(topic_t|document_d)*p(word_w|topic_t)$, which is essentially the probability that topic t generated word w.$ p(word_w | topic_t)$ = proportion of assignments to topic t over all documents that come from word w (how many w in all documents' words are assigned to t).$ p(topic_t | document_d)$ = proportion of words in document d that are assigned to topic t.To improve, for each document D, go through each word in D:.Because this is random, it will not be good.This gives random assignment of topic representations of all the documents and word distributions of all the topics.Given a set of documents, go through each document, and randomly assign each word in the document to one of the K topics.The algorithm uses collapsed Gibbs Sampling: Find set of topics that are most likely to have generated the collection of documents.if length is 5, then generate set of 5 words with probability distribution across words for a given topic such as "broccoli eat food animal meat" if select food topic, generate word 'broccoli' with 30\% probability and bananas with 15\% probability The generative process for each document w in corpus D is as belows: It assumes that the topics are generated before documents, and infer topics that could have generated the a corupus of documents (a review = a document). LDA is a type of Bayesian Inference Model. It then approximates probability distributions of topics in a given document and of words in a given topic.
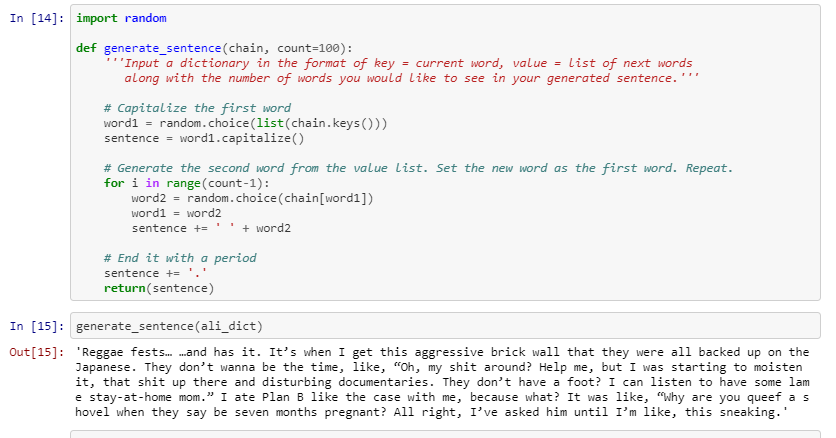
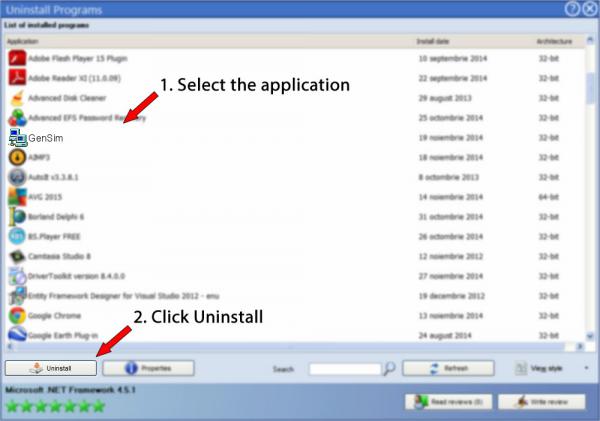
LDA assumes each document consists of a combination of topics, and each topic consists of a combination of words. A common method to quickly understand trends in topics being discussed in a corpus of text is Latent Dirichlet Allocation (LDA). Businesses can benefit immensely if they can understand general trends of what their customers are talking about online. With so many online reviews across many social media websites, it is hard for companies to keep track of their online reputation.
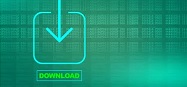